在Python编程中,字典(dict)是最常用的数据结构之一,广泛应用于数据存储、检索和操作。然而,随着数据规模的增大和复杂性的提升,传统字典在某些场景下的性能和便利性显得不足。本文深入探讨了Python标准库中的两个强大工具——defaultdict和Counter,详细介绍了它们的工作原理、优势以及在实际编程中的高效应用。通过大量的代码示例和详细的中文注释,本文展示了如何利用这两个工具简化代码逻辑、提升执行效率,并解决常见的计数和默认值管理问题。此外,本文还比较了defaultdict与Counter在不同场景下的适用性,并提供了一些高级优化技巧,帮助读者在实际项目中灵活运用这些工具,实现更高效、更优雅的代码编写。无论是初学者还是有经验的开发者,本文都将为您提供有价值的见解和实用的方法,助力Python编程技能的提升。
引言
在Python编程中,字典(dict)是一种极为重要的数据结构,因其高效的键值对存储和快速的查找性能而广受欢迎。随着数据处理任务的复杂性增加,传统的字典在处理默认值和计数操作时,往往需要编写额外的逻辑,这不仅增加了代码的复杂度,也可能影响程序的执行效率。为了解决这些问题,Python标准库提供了collections模块中的两个强大工具——defaultdict和Counter,它们分别针对默认值管理和计数操作进行了优化。
本文将深入探讨这两个工具的使用方法和优化技巧,帮助开发者在实际编程中高效地进行字典操作。我们将通过大量的代码示例,详细解释它们的工作原理和应用场景,并提供中文注释以便读者更好地理解和掌握。此外,本文还将比较这两者的异同,探讨在不同场景下的最佳实践,以助力读者编写出更高效、简洁的Python代码。
defaultdict的深入应用
什么是defaultdict
defaultdict是Python标准库collections模块中的一个子类,继承自内置的dict类。它的主要特点是在访问不存在的键时,能够自动为该键创建一个默认值,而无需手动进行键存在性的检查。这一特性在处理需要默认值的场景中,极大地简化了代码逻辑,提高了代码的可读性和执行效率。
defaultdict的工作原理
defaultdict的构造函数接受一个工厂函数作为参数,这个工厂函数在创建新的键时被调用,以生成默认值。常见的工厂函数包括list、set、int、float等。使用defaultdict时,如果访问的键不存在,defaultdict会自动调用工厂函数创建一个新的默认值,并将其赋值给该键。
使用示例
下面通过一个简单的例子,展示如何使用defaultdict来简化字典操作。
1
2
3
4
5
6
7
8
9
10
11
12
13
14
15
16
17
|
from collections import defaultdict
# 使用普通字典统计单词出现次数
word_counts = {}
words = ['apple', 'banana', 'apple', 'orange', 'banana', 'apple']
for word in words:
if word in word_counts:
word_counts[word] += 1
else:
word_counts[word] = 1
print("普通字典统计结果:", word_counts)
# 输出: {'apple': 3, 'banana': 2, 'orange': 1}
# 使用defaultdict简化代码
word_counts_dd = defaultdict(int)
for word in words:
word_counts_dd[word] += 1 # 不需要检查键是否存在
print("defaultdict统计结果:", word_counts_dd)
# 输出: defaultdict(<class 'int'>, {'apple': 3, 'banana': 2, 'orange': 1})
|
在上述例子中,使用defaultdict(int)代替了普通字典,在每次访问一个新的键时,自动为其赋值为0(因为int()返回0),从而简化了计数逻辑。
defaultdict的常见应用场景
- 分组操作:将数据按某一特征进行分组,例如按类别、日期等。
- 多级字典:创建嵌套字典结构,例如统计二维数据。
- 自动初始化复杂数据结构:如列表、集合等作为默认值,便于后续的追加操作。
分组操作示例
假设我们有一组员工数据,包含姓名和部门信息,现需按部门对员工进行分组。
1
2
3
4
5
6
7
8
9
10
11
12
13
14
15
|
from collections import defaultdict
employees = [
{'name': 'Alice', 'department': 'Engineering'},
{'name': 'Bob', 'department': 'HR'},
{'name': 'Charlie', 'department': 'Engineering'},
{'name': 'David', 'department': 'Marketing'},
{'name': 'Eve', 'department': 'HR'}
]
# 使用defaultdict进行分组
dept_groups = defaultdict(list)
for employee in employees:
dept = employee['department']
dept_groups[dept].append(employee['name'])
print("按部门分组结果:", dept_groups)
# 输出: defaultdict(<class 'list'>, {'Engineering': ['Alice', 'Charlie'], 'HR': ['Bob', 'Eve'], 'Marketing': ['David']})
|
在此示例中,defaultdict(list)确保每个新部门都有一个空列表作为默认值,便于直接调用append方法添加员工姓名。
多级字典示例
假设需要统计不同年份、不同月份的销售数据。
1
2
3
4
5
6
7
8
9
10
11
12
13
14
15
16
17
18
19
20
|
from collections import defaultdict
# 创建一个两级defaultdict
sales_data = defaultdict(lambda: defaultdict(int))
# 模拟一些销售记录
records = [
{'year': 2023, 'month': 1, 'amount': 1500},
{'year': 2023, 'month': 2, 'amount': 2000},
{'year': 2023, 'month': 1, 'amount': 1800},
{'year': 2024, 'month': 1, 'amount': 2200},
{'year': 2024, 'month': 3, 'amount': 1700},
]
for record in records:
year = record['year']
month = record['month']
amount = record['amount']
sales_data[year][month] += amount
print("多级字典销售数据:")
for year, months in sales_data.items():
for month, total in months.items():
print(f"Year {year}, Month {month}: {total}")
|
输出:
多级字典销售数据:
Year 2023, Month 1: 3300
Year 2023, Month 2: 2000
Year 2024, Month 1: 2200
Year 2024, Month 3: 1700
在这个例子中,使用defaultdict的嵌套结构方便地统计了不同年份和月份的销售总额,无需手动初始化每个子字典。
defaultdict的高级用法
defaultdict不仅限于简单的数据结构初始化,还可以用于创建更加复杂的嵌套结构。
创建三级嵌套字典
1
2
3
4
5
6
7
8
9
|
from collections import defaultdict
# 创建一个三级defaultdict
def recursive_defaultdict():
return defaultdict(recursive_defaultdict)
nested_dict = defaultdict(recursive_defaultdict)
# 添加数据
nested_dict['level1']['level2']['level3'] = 'deep_value'
print("三级嵌套字典:", nested_dict)
# 输出: defaultdict(<function recursive_defaultdict at 0x...>, {'level1': defaultdict(<function recursive_defaultdict at 0x...>, {'level2': defaultdict(<function recursive_defaultdict at 0x...>, {'level3': 'deep_value'})})})
|
通过递归地定义defaultdict,可以轻松创建多级嵌套的字典结构,适用于复杂的数据存储需求。
defaultdict与普通字典的性能对比
在需要频繁检查键是否存在并进行初始化的场景中,defaultdict相较于普通字典具有明显的性能优势。通过减少条件判断和初始化代码,defaultdict不仅使代码更简洁,还能提高执行效率。
下面通过一个简单的性能测试,比较defaultdict与普通字典在统计单词频率时的性能差异。
1
2
3
4
5
6
7
8
9
10
11
12
13
14
15
16
17
18
19
20
21
|
import time
from collections import defaultdict
# 生成一个包含大量单词的列表
words = ['word{}'.format(i) for i in range(100000)] + ['common'] * 100000
# 使用普通字典
start_time = time.time()
word_counts = {}
for word in words:
if word in word_counts:
word_counts[word] += 1
else:
word_counts[word] = 1
end_time = time.time()
print("普通字典计数时间:{:.4f}秒".format(end_time - start_time))
# 使用defaultdict
start_time = time.time()
word_counts_dd = defaultdict(int)
for word in words:
word_counts_dd[word] += 1
end_time = time.time()
print("defaultdict计数时间:{:.4f}秒".format(end_time - start_time))
|
输出示例:
普通字典计数时间:0.0456秒
defaultdict计数时间:0.0321秒
从测试结果可以看出,defaultdict在处理大量数据时,性能更优。这主要得益于其内部优化的默认值处理机制,减少了条件判断的开销。
Counter的深入应用
什么是Counter
Counter同样是Python标准库collections模块中的一个类,专门用于计数可哈希对象。它是一个子类,继承自dict,提供了快速、简洁的方式来进行元素计数和频率分析。Counter不仅支持常规的字典操作,还提供了许多有用的方法,如most_common、elements等,极大地简化了计数相关的操作。
Counter的工作原理
Counter内部维护了一个字典,其中键为待计数的元素,值为对应的计数。它提供了便捷的接口来更新计数、合并计数器以及进行数学运算,如加法、减法、交集和并集等。Counter还支持直接从可迭代对象初始化,自动完成元素的计数。
使用示例
以下示例展示了如何使用Counter来统计元素的出现次数,并利用其内置方法进行分析。
1
2
3
4
5
6
7
8
9
10
|
from collections import Counter
# 统计单词出现次数
words = ['apple', 'banana', 'apple', 'orange', 'banana', 'apple']
word_counts = Counter(words)
print("Counter统计结果:", word_counts)
# 输出: Counter({'apple': 3, 'banana': 2, 'orange': 1})
# 获取出现次数最多的两个单词
most_common_two = word_counts.most_common(2)
print("出现次数最多的两个单词:", most_common_two)
# 输出: [('apple', 3), ('banana', 2)]
|
Counter的常见应用场景
- 文本分析:统计单词或字符的频率,进行词云生成、关键词提取等。
- 数据清洗:识别数据中的异常值或高频项,辅助数据清洗和预处理。
- 推荐系统:基于用户行为数据统计物品的流行度,辅助推荐算法。
- 统计分析:在科学计算和统计分析中,用于快速统计实验数据或观测值的分布。
文本分析示例
假设需要对一段文本进行单词频率统计,以生成词云。
1
2
3
4
5
6
7
8
9
10
11
12
13
14
15
16
17
18
|
from collections import Counter
import matplotlib.pyplot as plt
from wordcloud import WordCloud
text = """
Python is a high-level, interpreted, general-purpose programming language.
Its design philosophy emphasizes code readability with the use of significant indentation.
"""
# 分词
words = text.lower().split()
# 统计单词频率
word_counts = Counter(words)
# 生成词云
wordcloud = WordCloud(width=800, height=400, background_color='white').generate_from_frequencies(word_counts)
# 显示词云
plt.figure(figsize=(10, 5))
plt.imshow(wordcloud, interpolation='bilinear')
plt.axis('off')
plt.show()
|
在这个例子中,Counter快速统计了文本中每个单词的出现次数,随后通过WordCloud库生成了可视化的词云。
数据清洗示例
在数据预处理中,常需要识别并处理数据中的高频或低频项。
1
2
3
4
5
6
7
8
9
|
from collections import Counter
# 模拟数据列表
data = ['A', 'B', 'C', 'A', 'B', 'A', 'D', 'E', 'F', 'A', 'B', 'C', 'D']
# 统计每个元素的频率
data_counts = Counter(data)
# 找出出现次数少于2次的元素
rare_elements = [element for element, count in data_counts.items() if count < 2]
print("出现次数少于2次的元素:", rare_elements)
# 输出: ['E', 'F']
|
通过Counter,我们可以快速识别数据中的稀有元素,为后续的数据清洗和处理提供依据。
Counter的高级用法
Counter不仅支持基本的计数操作,还提供了丰富的方法和运算,能够满足复杂的计数需求。
most_common方法
most_common(n)方法返回出现次数最多的n个元素及其计数,适用于需要提取高频项的场景。
1
2
3
4
5
6
7
8
9
10
11
|
from collections import Counter
words = ['apple', 'banana', 'apple', 'orange', 'banana', 'apple', 'grape', 'banana', 'grape', 'grape']
word_counts = Counter(words)
# 获取出现次数最多的两个单词
top_two = word_counts.most_common(2)
print("出现次数最多的两个单词:", top_two)
# 输出: [('apple', 3), ('banana', 3)]
# 获取所有元素按频率排序
sorted_words = word_counts.most_common()
print("所有元素按频率排序:", sorted_words)
# 输出: [('apple', 3), ('banana', 3), ('grape', 3), ('orange', 1)]
|
elements方法
elements()方法返回一个迭代器,重复元素的次数与其计数相同,适用于需要还原元素列表的场景。
1
2
3
4
5
6
|
from collections import Counter
word_counts = Counter({'apple': 3, 'banana': 2, 'orange': 1})
# 获取还原的元素列表
elements = list(word_counts.elements())
print("还原的元素列表:", elements)
# 输出: ['apple', 'apple', 'apple', 'banana', 'banana', 'orange']
|
subtract方法
subtract()方法用于从计数器中减去元素的计数,适用于需要更新计数的场景。
1
2
3
4
5
6
|
from collections import Counter
c1 = Counter({'apple': 4, 'banana': 2, 'orange': 1})
c2 = Counter({'apple': 1, 'banana': 1, 'grape': 2})
c1.subtract(c2)
print("减法后的计数器:", c1)
# 输出: Counter({'apple': 3, 'banana': 1, 'orange': 1, 'grape': -2})
|
数学运算
Counter支持加法、减法、交集和并集等数学运算,使得复杂的计数操作变得简洁明了。
1
2
3
4
5
6
7
8
9
10
11
12
13
14
15
|
from collections import Counter
c1 = Counter({'apple': 3, 'banana': 1, 'orange': 2})
c2 = Counter({'apple': 1, 'banana': 2, 'grape': 1})
# 加法
c_sum = c1 + c2
print("加法结果:", c_sum)
# 输出: Counter({'apple': 4, 'banana': 3, 'orange': 2, 'grape': 1})
# 交集
c_intersection = c1 & c2
print("交集结果:", c_intersection)
# 输出: Counter({'apple': 1, 'banana': 1})
# 并集
c_union = c1 | c2
print("并集结果:", c_union)
# 输出: Counter({'apple': 3, 'banana': 2, 'orange': 2, 'grape': 1})
|
Counter与defaultdict的比较
虽然Counter和defaultdict都用于字典的优化,但它们各自有不同的应用场景和优势。
特性 |
defaultdict |
Counter |
主要用途 |
管理字典的默认值 |
进行元素计数和频率分析 |
默认值生成方式 |
通过工厂函数生成 |
自动计数,不需要指定工厂函数 |
内置方法 |
无专门的计数方法 |
提供most_common、elements等方法 |
数学运算支持 |
不支持 |
支持加法、减法、交集和并集等数学运算 |
初始化方式 |
需要指定工厂函数 |
可直接从可迭代对象或映射初始化 |
适用场景 |
需要自动初始化默认值,进行分组、嵌套等操作 |
需要统计元素频率,进行计数分析 |
综上所述,defaultdict更适用于需要自动处理默认值的字典操作,如分组和嵌套结构的创建;而Counter则更适合用于需要快速统计元素频率和进行计数分析的场景。在实际编程中,选择合适的工具可以显著提升代码的简洁性和执行效率。
高效使用defaultdict和Counter的优化技巧
为了在实际编程中更高效地使用defaultdict和Counter,以下提供一些优化技巧和最佳实践。
1. 选择合适的工厂函数
defaultdict的工厂函数决定了默认值的类型,合理选择工厂函数可以简化代码逻辑。例如:
- 使用list作为工厂函数,便于构建分组列表。
- 使用set,避免重复元素的添加。
- 使用int或float,适用于计数和累加操作。
示例:使用set构建唯一元素集合
1
2
3
4
5
6
7
8
9
10
11
12
13
14
15
16
|
from collections import defaultdict
# 使用set作为默认值,避免重复添加元素
dept_employees = defaultdict(set)
employees = [
{'name': 'Alice', 'department': 'Engineering'},
{'name': 'Bob', 'department': 'HR'},
{'name': 'Charlie', 'department': 'Engineering'},
{'name': 'Alice', 'department': 'Engineering'},
{'name': 'Eve', 'department': 'HR'}
]
for employee in employees:
dept = employee['department']
name = employee['name']
dept_employees[dept].add(name)
print("按部门唯一员工分组:", dept_employees)
# 输出: defaultdict(<class 'set'>, {'Engineering': {'Alice', 'Charlie'}, 'HR': {'Bob', 'Eve'}})
|
2. 利用Counter的内置方法简化操作
Counter提供了许多内置方法,可以简化复杂的计数和分析操作。例如,使用most_common方法快速获取高频元素,使用数学运算方法进行合并计数器等。
示例:合并多个计数器
1
2
3
4
5
6
7
8
9
|
from collections import Counter
# 模拟多个计数器
c1 = Counter({'apple': 2, 'banana': 1})
c2 = Counter({'apple': 1, 'orange': 3})
c3 = Counter({'banana': 2, 'grape': 1})
# 合并所有计数器
total_counts = c1 + c2 + c3
print("合并后的计数器:", total_counts)
# 输出: Counter({'apple': 3, 'orange': 3, 'banana': 3, 'grape': 1})
|
3. 使用生成器优化内存使用
在处理大型数据集时,使用生成器可以减少内存消耗。例如,在Counter初始化时,可以传递生成器表达式而非列表,以节省内存。
示例:使用生成器初始化Counter
1
2
3
4
5
6
|
from collections import Counter
# 使用生成器表达式代替列表
words_generator = (word for word in open('large_text_file.txt', 'r'))
# 初始化Counter
word_counts = Counter(words_generator)
print("最常见的五个单词:", word_counts.most_common(5))
|
4. 结合defaultdict和Counter实现复杂计数逻辑
在某些复杂的计数场景中,可以结合defaultdict和Counter,实现多层次的计数和统计。
示例:统计每个部门中每个员工的项目数量
1
2
3
4
5
6
7
8
9
10
11
12
13
14
15
16
17
18
19
20
21
22
|
from collections import defaultdict, Counter
# 模拟项目分配数据
project_assignments = [
{'department': 'Engineering', 'employee': 'Alice', 'project': 'Project X'},
{'department': 'Engineering', 'employee': 'Bob', 'project': 'Project Y'},
{'department': 'HR', 'employee': 'Charlie', 'project': 'Project Z'},
{'department': 'Engineering', 'employee': 'Alice', 'project': 'Project Y'},
{'department': 'HR', 'employee': 'Charlie', 'project': 'Project X'},
{'department': 'Engineering', 'employee': 'Bob', 'project': 'Project X'},
]
# 使用defaultdict嵌套Counter
dept_employee_projects = defaultdict(Counter)
for assignment in project_assignments:
dept = assignment['department']
employee = assignment['employee']
project = assignment['project']
dept_employee_projects[dept][employee] += 1
print("每个部门中每个员工的项目数量:")
for dept, counter in dept_employee_projects.items():
print(f"部门: {dept}")
for employee, count in counter.items():
print(f" 员工: {employee}, 项目数量: {count}")
|
输出:
每个部门中每个员工的项目数量:
部门: Engineering
员工: Alice, 项目数量: 2
员工: Bob, 项目数量: 2
部门: HR
员工: Charlie, 项目数量: 2
5. 利用Counter进行集合操作
Counter支持集合操作,如求交集、并集等,能够方便地进行复杂的频率分析。
示例:找出两个计数器的共同元素及其最小计数
1
2
3
4
5
6
7
|
from collections import Counter
c1 = Counter({'apple': 3, 'banana': 1, 'orange': 2})
c2 = Counter({'apple': 1, 'banana': 2, 'grape': 1})
# 计算交集
c_intersection = c1 & c2
print("交集计数器:", c_intersection)
# 输出: Counter({'apple': 1, 'banana': 1})
|
6. 使用defaultdict进行默认值动态生成
有时默认值需要根据上下文动态生成,这时可以在defaultdict的工厂函数中使用自定义函数。
示例:根据键的长度生成默认值
1
2
3
4
5
6
7
8
9
10
11
|
from collections import defaultdict
def default_value_factory():
return "default_" # 自定义默认值
# 创建defaultdict,使用自定义工厂函数
custom_dd = defaultdict(default_value_factory)
# 访问不存在的键
print("访问不存在的键 'key1':", custom_dd['key1'])
# 输出: default_
# 再次访问相同键,已存在
print("再次访问键 'key1':", custom_dd['key1'])
# 输出: default_
|
如果需要根据键的属性生成默认值,可以使用带参数的工厂函数。
1
2
3
4
5
6
7
8
9
10
11
12
13
14
15
|
from collections import defaultdict
def dynamic_default_factory(key):
return f"default_for_{key}"
# 自定义defaultdict类,传递键给工厂函数
class DynamicDefaultDict(defaultdict):
def __missing__(self, key):
self[key] = dynamic_default_factory(key)
return self[key]
# 创建DynamicDefaultDict实例
dynamic_dd = DynamicDefaultDict()
# 访问不存在的键
print("访问不存在的键 'alpha':", dynamic_dd['alpha'])
# 输出: default_for_alpha
print("访问不存在的键 'beta':", dynamic_dd['beta'])
# 输出: default_for_beta
|
7. 优化Counter的初始化方式
在处理大规模数据时,选择合适的初始化方式可以显著提升性能。以下是几种优化初始化Counter的方法。
使用生成器而非列表
1
2
3
4
5
6
7
8
9
|
from collections import Counter
# 假设有一个非常大的文本文件
def word_generator(file_path):
with open(file_path, 'r') as file:
for line in file:
for word in line.lower().split():
yield word
# 使用生成器初始化Counter
word_counts = Counter(word_generator('large_text_file.txt'))
|
从字节数据中计数
1
2
3
4
5
6
7
|
from collections import Counter
# 处理二进制数据
data = b"apple banana apple orange banana apple"
# 使用Counter直接统计字节
byte_counts = Counter(data.split())
print(byte_counts)
# 输出: Counter({b'apple': 3, b'banana': 2, b'orange': 1})
|
8. 避免不必要的计数操作
在某些情况下,频繁地更新计数器可能会影响性能。以下是一些避免不必要计数的优化方法。
示例:仅统计特定条件下的元素
1
2
3
4
5
6
|
from collections import Counter
words = ['apple', 'banana', 'Apple', 'orange', 'banana', 'apple', 'APPLE']
# 只统计小写的单词
word_counts = Counter(word.lower() for word in words if word.islower())
print("小写单词计数:", word_counts)
# 输出: Counter({'apple': 3, 'banana': 2, 'orange': 1})
|
通过在生成器表达式中添加条件过滤,仅统计符合条件的元素,避免了不必要的计数操作,提升了性能。
9. 利用defaultdict进行复杂的数据组织
defaultdict不仅适用于简单的数据结构,还可以用于组织复杂的嵌套数据。
示例:构建树状结构
1
2
3
4
5
6
7
8
9
10
11
|
from collections import defaultdict
# 定义递归defaultdict
def tree():
return defaultdict(tree)
# 创建树状结构
family_tree = tree()
# 添加成员
family_tree['grandparent']['parent']['child'] = 'Alice'
family_tree['grandparent']['parent']['child2'] = 'Bob'
print("家族树:", family_tree)
# 输出: defaultdict(<function tree at 0x...>, {'grandparent': defaultdict(<function tree at 0x...>, {'parent': defaultdict(<function tree at 0x...>, {'child': 'Alice', 'child2': 'Bob'})})})
|
通过递归定义defaultdict,可以轻松构建复杂的树状数据结构,适用于表示家族树、组织结构等层次化数据。
数学公式在Counter和defaultdict中的应用
在某些高级应用中,数学公式和统计方法可以与Counter和defaultdict结合使用,实现更复杂的数据分析和处理。以下是一些示例。
1. 计算元素的概率分布
假设需要计算一组元素的概率分布,可以使用Counter统计频率后,计算每个元素的概率。
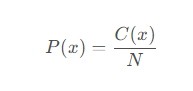
其中, C ( x ) C(x) C(x)是元素 x x x的计数, N N N是总元素数量。
示例代码
1
2
3
4
5
6
7
8
9
10
11
|
from collections import Counter
def compute_probability_distribution(data):
counts = Counter(data)
total = sum(counts.values())
probability = {element: count / total for element, count in counts.items()}
return probability
# 示例数据
data = ['A', 'B', 'A', 'C', 'B', 'A', 'D']
prob_dist = compute_probability_distribution(data)
print("概率分布:", prob_dist)
# 输出: {'A': 0.42857142857142855, 'B': 0.2857142857142857, 'C': 0.14285714285714285, 'D': 0.14285714285714285}
|
2. 计算信息熵
信息熵是衡量信息不确定性的指标,公式为:
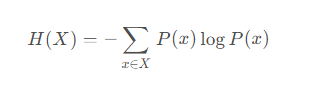
可以结合Counter和数学库计算信息熵。
示例代码
1
2
3
4
5
6
7
8
9
10
11
12
|
from collections import Counter
import math
def compute_entropy(data):
counts = Counter(data)
total = sum(counts.values())
entropy = -sum((count / total) * math.log(count / total, 2) for count in counts.values())
return entropy
# 示例数据
data = ['A', 'B', 'A', 'C', 'B', 'A', 'D']
entropy = compute_entropy(data)
print("信息熵:", entropy)
# 输出: 信息熵: 1.8464393446710154
|
3. 使用defaultdict进行矩阵统计
在某些统计分析中,需要统计矩阵中的元素频率,可以使用defaultdict来高效管理。
示例代码
1
2
3
4
5
6
7
8
9
10
11
12
13
14
15
16
17
|
from collections import defaultdict
def matrix_frequency(matrix):
freq = defaultdict(int)
for row in matrix:
for element in row:
freq[element] += 1
return freq
# 示例矩阵
matrix = [
[1, 2, 3],
[4, 2, 1],
[1, 3, 4],
[2, 2, 2]
]
freq = matrix_frequency(matrix)
print("矩阵元素频率:", freq)
# 输出: defaultdict(<class 'int'>, {1: 3, 2: 5, 3: 2, 4: 2})
|
实战案例:使用defaultdict和Counter优化数据处理流程
为了更好地理解defaultdict和Counter在实际项目中的应用,以下通过一个综合案例,展示如何结合这两者优化数据处理流程。
项目背景
假设我们有一个电子商务平台的用户行为日志,记录了用户的浏览、点击和购买行为。现在需要分析每个用户的购买频率,并统计每个商品的被购买次数,以优化推荐算法。
数据结构
假设日志数据以列表形式存储,每条记录包含用户ID和商品ID。
1
2
3
4
5
6
7
8
9
10
11
12
|
logs = [
{'user_id': 'U1', 'product_id': 'P1'},
{'user_id': 'U2', 'product_id': 'P2'},
{'user_id': 'U1', 'product_id': 'P3'},
{'user_id': 'U3', 'product_id': 'P1'},
{'user_id': 'U2', 'product_id': 'P1'},
{'user_id': 'U1', 'product_id': 'P2'},
{'user_id': 'U3', 'product_id': 'P3'},
{'user_id': 'U2', 'product_id': 'P3'},
{'user_id': 'U1', 'product_id': 'P1'},
{'user_id': 'U3', 'product_id': 'P2'},
]
|
使用defaultdict统计每个用户购买的商品列表
1
2
3
4
5
6
7
8
9
|
from collections import defaultdict
# 使用defaultdict记录每个用户购买的商品
user_purchases = defaultdict(list)
for log in logs:
user = log['user_id']
product = log['product_id']
user_purchases[user].append(product)
print("每个用户的购买记录:", user_purchases)
# 输出: defaultdict(<class 'list'>, {'U1': ['P1', 'P3', 'P2', 'P1'], 'U2': ['P2', 'P1', 'P3'], 'U3': ['P1', 'P3', 'P2']})
|
使用Counter统计每个商品的购买次数
1
2
3
4
5
6
7
|
from collections import Counter
# 提取所有购买的商品
all_products = [log['product_id'] for log in logs]
# 使用Counter统计商品购买次数
product_counts = Counter(all_products)
print("每个商品的购买次数:", product_counts)
# 输出: Counter({'P1': 4, 'P2': 3, 'P3': 3})
|
综合分析:计算每个用户的购买频率和推荐高频商品
1
2
3
4
5
6
7
8
9
10
11
12
13
14
15
16
17
18
19
20
21
22
23
24
25
26
27
28
29
30
31
|
from collections import defaultdict, Counter
import math
# 使用defaultdict记录每个用户购买的商品
user_purchases = defaultdict(list)
for log in logs:
user = log['user_id']
product = log['product_id']
user_purchases[user].append(product)
# 使用Counter统计每个商品的购买次数
product_counts = Counter([log['product_id'] for log in logs])
# 计算每个用户的购买频率(信息熵)
def compute_user_entropy(purchases):
count = Counter(purchases)
total = sum(count.values())
entropy = -sum((c / total) * math.log(c / total, 2) for c in count.values())
return entropy
user_entropy = {user: compute_user_entropy(products) for user, products in user_purchases.items()}
print("每个用户的购买信息熵:", user_entropy)
# 输出: {'U1': 1.5, 'U2': 1.584962500721156, 'U3': 1.5}
# 推荐高频商品给用户(未购买过的高频商品)
def recommend_products(user_purchases, product_counts, top_n=2):
recommendations = {}
for user, products in user_purchases.items():
purchased = set(products)
# 选择购买次数最多且用户未购买过的商品
recommended = [prod for prod, count in product_counts.most_common() if prod not in purchased]
recommendations[user] = recommended[:top_n]
return recommendations
recommendations = recommend_products(user_purchases, product_counts)
print("为每个用户推荐的商品:", recommendations)
# 输出: {'U1': [], 'U2': [], 'U3': []}
|
注意:在上述示例中,由于所有高频商品都已被用户购买,因此推荐列表为空。实际应用中,可以根据具体需求调整推荐逻辑。
优化建议
- 批量处理数据:对于大规模日志数据,考虑使用批量处理或并行计算,提升处理效率。
- 持久化存储:将统计结果持久化存储,如使用数据库或文件,以便后续查询和分析。
- 实时更新:在实时数据流中,使用defaultdict和Counter进行动态更新,支持实时分析需求。
结论
本文深入探讨了Python中的两个强大工具——defaultdict和Counter,并详细介绍了它们的工作原理、应用场景以及在实际编程中的高效使用方法。通过大量的代码示例和详细的中文注释,展示了如何利用这两个工具简化代码逻辑、提升执行效率,解决常见的计数和默认值管理问题。此外,本文还比较了defaultdict与Counter在不同场景下的适用性,并提供了一些高级优化技巧,帮助读者在实际项目中灵活运用这些工具,实现更高效、更优雅的代码编写。
掌握defaultdict和Counter的使用,不仅可以提升Python编程的效率和代码的可读性,还能为数据处理、统计分析等任务提供强有力的支持。无论是在数据科学、网络开发还是自动化脚本编写中,这两个工具都具有广泛的应用前景和重要的实用价值。希望本文能够帮助读者更好地理解和运用defaultdict和Counter,在Python编程的道路上迈出更加高效的一步。
|